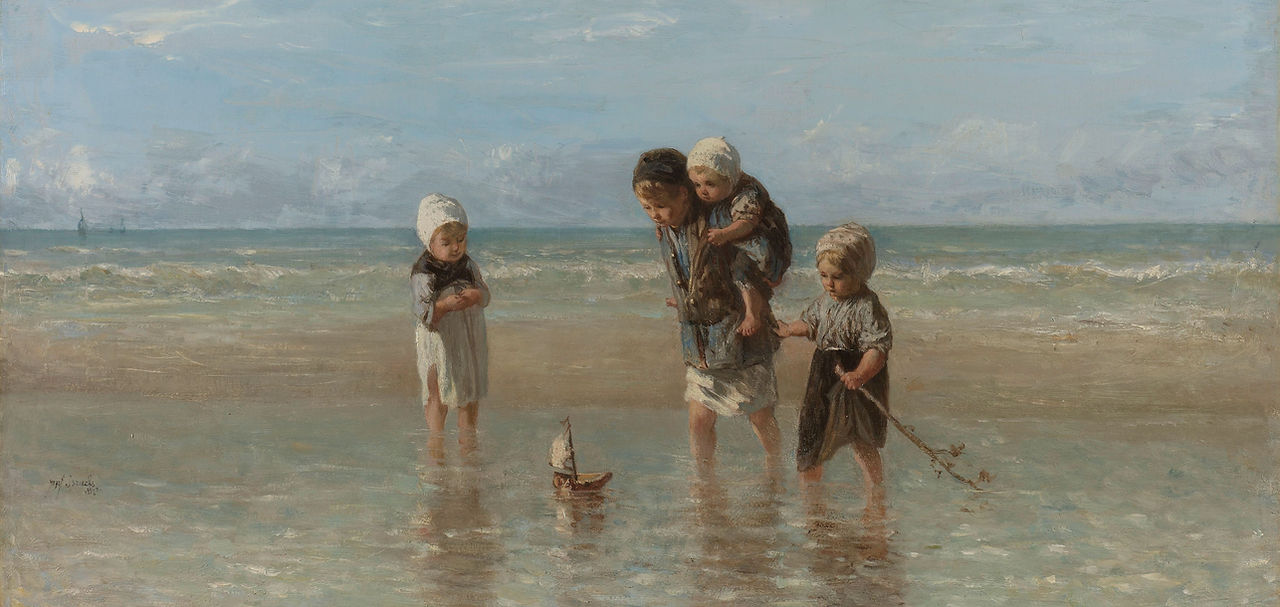
Causal AI for Personalized Interventions
Using causal AI to optimize and personalize interventions in medicine and public health to make them more cost effective.
Bargagli Stoffi, F.J., Tortù, C., Forastiere, L. Heterogeneous Treatment and Spillover Effects under Clustered Network Interference. Annals of Applied Statistics, 19(1), 28-55, 2025.
[paper]
Zorzetto, D., Bargagli Stoffi, F.J., Canale, A., Dominici, F. Confounder-Dependent Bayesian Mixture Model: Characterizing Heterogeneity of Causal Effects in Air Pollution Epidemiology. Biometrics, 80(2), 2024.
Bargagli Stoffi, F. J., De Witte, K., Gnecco. G. Heterogeneous Causal Effects with Imperfect Compliance: a Bayesian Machine Learning Approach. Annals of Applied Statistics, 16 (3), 1986-2009, 2022.
[paper] Coverage: [R-bloggers post] [YoungStats post]​
Zorzetto, D., Canale, A., Mealli, F., Dominici, F., Bargagli Stoffi, F.J., Bayesian Nonparametrics for Principal Statification with Continuous Post-Treatment Variables arXiv preprint arXiv:2302.11656.
[preprint]
Bargagli Stoffi, F.J., Gnecco, G. Causal Tree with Instrumental Variable: An Extension of the Causal Tree Framework to Irregular Assignment Mechanisms. International Journal of Data Science and Analytics, 9, 315–337, 2020.
[paper]
Image: “Children of the Sea", Jozef Israels, 1872. Rijksmuseum, Amsterdam, The Netherlands.